
Exclusive Interview with PharmaShots: Moshe Safran of RSIP Vision Share Insight on the 3D Reconstruction Technology
In an interview with PharmaShots, Moshe Safran, CEO at RSIP Vision shared his views on the new coronary module in the complete 3D reconstruction of the coronary vasculature during angiography
Shots:
- The company reported the 3D reconstruction technology that focused on AI to generate 3D models of patient anatomy from 2D images. The purpose of the technology is to create precise, personalized interventions
- Machine learning can be used for better-compressed sensing & accelerating the acquisition time. The technology is used in a variety of medical modalities
- For cardiology, the company generates a 3D reconstruction of 2D of cardiac chambers, vessels, coronary arteries from 2D images using neural networks & classical computer vision or delineates cardiac structures through static & dynamic imaging. For orthopedics, 3D bone models from 2D X-rays & detect existing metals within the joints
Tuba: What 3D reconstruction looks like, and how does it benefit the practitioner?
Moshe Safran: When we talk about 3D reconstruction there are actually two main vectors.
The first one is using two or three X-rays to create a full 3D model of a particular type of anatomy, for instance, the knee or the hip. This is very important for precision orthopedic surgeries, both for robotic use cases and for patient-specific solutions where a 3D model is needed. You can get this from a CT scan, but that involves increased radiation, friction in the workflow, and even more critically there are very high barriers to reimbursement for CTs in the U.S. for these procedures. This means that being able to use 2D X-rays for 3D planning provides accessibility to a huge segment of patients who otherwise would not be able to benefit from these types of treatments. Going forward, we think this technology has even wider potential to significantly reduce the radiation doses for many use cases where a 3D image is required which currently entails a full CT.
The second vector of 3D reconstruction involves endoscopic videos. Understanding the depth in these scenes, and doing it well, is a significant challenge that is being very actively pursued in both academia and industry and will open up a lot of cool Artificial Intelligence (AI)-based “driver assist” kind of features based on improved solutions for this challenging task.
At RSIP Vision, our 2D-to-3D reconstruction technology trains AI to generate 3D models of patient anatomy from two-dimensional images. We’re at quite an advanced point in the first couple of applications, and we’re seeing how useful this technology will be across the board for a wide variety of anatomical areas, images, and procedures. Our aim for this technology is to create precise, personalized interventions that can only be accomplished with 3D information while making the information accessible to many patients. Oftentimes, only 2D imaging is available whether for reasons of cost, reimbursement, or due to the desire to limit exposure to radiation — so RSIP Vision is working to help provide the most accurate treatment plans in these situations.
Tuba: Why is it more important than ever to incorporate AI in healthcare/MedTech?
Moshe Safran: AI and machine learning technology have already been shown to have a tremendous benefit across a variety of points of care. The opportunities are endless, and the technology will only serve to exponentially improve. Learning algorithms offer significant advantages for clinical decision-making because they continue to become more precise and fine-tuned as they encounter more and more data, providing a huge boost for diagnostic capabilities, care processes, and treatment options — which propels the two main goals of improving patient outcomes while reducing on care costs at the same time. Radiological image analysis, as well as digital pathology, are two examples that come to mind as the most currently widespread applications. These spaces had an early advantage due to the wide availability of copious amounts of data.
The surgical and interventional space is also in our view as the up-and-coming area of focus, which is why we choose to focus our efforts primarily there. The entire surgical robotics industry is racing to integrate AI and use it to differentiate their products beyond the hardware itself. These use cases are generating tremendous interest and investment and come with their own challenges, requiring a very high degree of creativity and multidisciplinary expertise. Seeing the high level of effort being put into these applications in the industry, some of these efforts will definitely come to fruition, leading to better tools to assist the surgeons in fulfilling their calling. Contrary to popular belief, it’s not about trying to replace or automate what surgeons do best. Rather - it’s about giving them better and more precise tools and reducing their cognitive load. For the system as a whole, we want to provide better efficiency and better accessibility to treatment. So, it’s important to always focus on what is the benefit of a particular technology, what problem is it solving, and how we can manage the innovation process to fulfill the potential and get it into the hands of the users.
We're just at the tip of the iceberg. There has already been a lot of success in radiology, reducing the workload by automating measurements. Companies like viz.ai are providing alerts faster than the healthcare system can manually process the information regarding digital pathology. A ton of data has been amassed and they are going mainstream. But underneath the surface, there is a huge activity in the medical device industry to integrate more computer vision and AI for the procedures themselves, like for surgical robotics, orthopedic surgery, endoscopic, and minimally invasive procedures. The road to the OR is sometimes longer but the value is going to be tremendous.
Tuba: How do AI algorithms improve visualization while reducing the number of images needed to capture the full picture?
Moshe Safran: By using automated algorithms, we are essentially trying to replicate what an expert would with a particular image — which interprets it accurately based on what they know about the targeted area of interest. Many of our products are designed to determine the precise measurements of the target areas that images capture, but not all physicians in all hospital environments carry the same experience in interpreting that specific type of image or lack the expertise of a particular area. Ultrasound images, for example, are very noisy and hard to interpret, and the edges often disappear. Our software can automatically measure and show the user where the specific point of interest is and provide a precise measurement of it. What AI and machine learning allow us to do is teach the computer to learn from examples. Instead of writing specific hand-crafted algorithms, we provide labeled examples and show the machine-learning system examples of the inputs/outputs that it should be generating, and as more and more data is fed into the system, it learns how it should be processing these images. You start with a general-purpose system that takes images and through many examples, it eventually learns the details of what it should be doing with an image all on its own. This of course requires a wealth of imaging data to be utilized to ensure the algorithm is being properly trained based on previous interpretations from experts.
With AI, we can use the wealth of images coming from the C-arm, or the camera, and compare those to the patient’s anatomy and to the plan to help the surgeon visualize exactly what is going on. In many cases, we can take a 2D image and construct a 3D from it, using AI to elevate image capture and as a result, use fewer projections in an intra-op CT. Machine-learning can also be used to do better-compressed sensing or to accelerate the acquisition time for an example of an MRI by teaching the computer to reconstruct the scene from partial and limited information.
Tuba: Would you like to describe the RSIP Vision’s work in different fields?
Moshe Safran: Our technology is used across a variety of medical modalities. In the last year alone, we've developed new technology impacting the fields of Cardiology, Orthopedics, Pulmonology, Ophthalmology, Urology, Surgical Robots, and more. When it comes to cardiology, create a 3D reconstruction of 2D of cardiac chambers, great vessels, and coronary arteries from 2D images using neural networks and classical computer vision or delineate cardiac structures through static and dynamic imaging. For orthopedics, we can generate 3D bone models from 2D X-rays, and even detect existing metals within the joints. Over the last year, we've developed a new technology that assists physicians across all these fields to make quicker and more confident treatment decisions.
Tuba: Are you looking for partners or any collaborations to widespread the adoption of this technology?
Moshe Safran: Yes, we collaborate closely with the medical device industry and continue to seek strategic partners to take our newest technologies to market.
Tuba: What makes you work on the application of computer vision in medical imaging?
Moshe Safran: We’re enabling physicians to do their work better — diagnosing, planning, treating with ease and precision — by creating an ever-widening scope of medical applications, breakthrough solutions, and customizable modules ultimately improving patient outcomes
Tuba: What next can we expect from the RSIP vision in the next 12 months?
Moshe Safran: We’re continuing down the road of elevated planning and creating full products with regulatory approval. In addition, precise intra-op and intra-procedural navigation are very much on our radar and you can expect some interesting developments on this front for 2022 as well.
About Author:
Moshe Safran is the CEO of RSIP Vision. He is an experienced R&D leader in computer vision algorithm development. He earned a bachelor's degree in B.Sc. Physics from the Hebrew University of Jerusalem and Computational Neuroscience from the same university
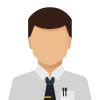
This content piece was prepared by our former Senior Editor. She had expertise in life science research and was an avid reader. For any query reach out to us at connect@pharmashots.com